< Back
Everything You Need to Know About Using AI For Research
Declan Gessel
Sep 6, 2024
With college assignment deadlines looming, you sit down to start an essay. You reach for your notes and realize they could be more organized and helpful. You open your laptop and stare at a blank document while dreading the research process. Instead of writing, you’ll have to find sources for the essay to get started. To make matters worse, using sources from a decade ago does not excite you. You need something fresher and more relevant to today’s world. You need a quick and easy way to find sources for your essay.
That’s where AI tools come in to find sources for essay. The intelligent algorithms can help you quickly locate the most relevant and up-to-date sources for your assignment so you can finish your essay and move on to your next task. This guide will help you understand how using AI for research can ease essay writing. One of the most valuable AI tools for research is Jotbot’s source finder. This tool quickly finds relevant academic sources for your essay, so you can spend less time researching and more time writing.
Table Of Contents
What is AI for Research?
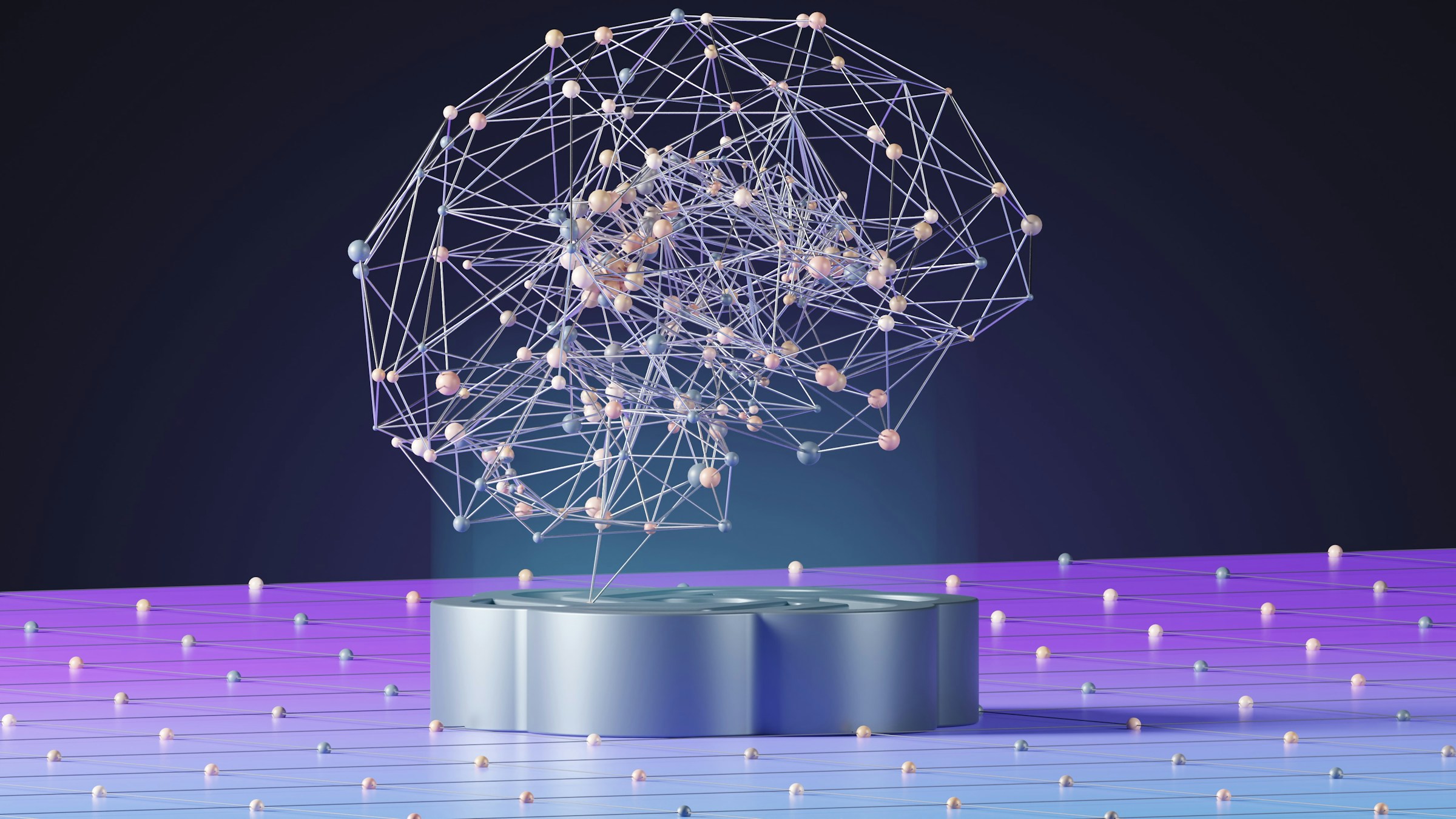
AI, or artificial intelligence, has revolutionized numerous industries, and research is no exception. When applied to research, AI offers a powerful toolkit for scholars to enhance their work, accelerate discoveries, and gain deeper insights.
Enhanced Data Analysis: Discover What You’ve Been Missing
AI algorithms can process vast amounts of data with incredible speed and accuracy, identifying patterns, trends, and anomalies that may be difficult for humans to detect. This enables researchers to uncover hidden insights and make more informed conclusions.
Automation of Repetitive Tasks: Free Up Your Brain for More Complex Work
AI can automate routine tasks, such as data cleaning, preprocessing, and analysis, freeing up researchers to focus on more complex and creative aspects of their work. This increased efficiency can lead to faster research cycles and higher productivity.
Generation of New Hypotheses: Let the Machines Help You Discover the Next Big Thing
AI can generate new hypotheses based on data analysis, stimulating researchers to explore novel research directions and potentially make groundbreaking discoveries.
Improved Collaboration: Facilitate Interdisciplinary Research with AI
AI-powered tools can facilitate collaboration among researchers, enabling them to share data, insights, and resources more effectively. This can lead to more interdisciplinary research and accelerated progress.
Ethical Considerations: Use AI Responsibly to Avoid Research Biases
While AI offers numerous benefits, the ethical implications of its use in research must be considered. Researchers must ensure that AI is used responsibly and ethically, avoiding biases and ensuring data privacy.
Applications of AI in Research
Natural Language Processing (NLP)
AI can be used to analyze text data, such as research papers, patents, and social media posts, to extract critical information, identify trends, and understand sentiment.
Machine Learning
Machine learning algorithms can build predictive models, classify data, and identify patterns in complex datasets. This is particularly useful in biology, medicine, and social sciences.
Data Visualization
AI can help researchers visualize large and complex datasets meaningfully, making it easier to understand trends and communicate findings.
Simulation and Modeling
AI can create simulations and models of complex systems, such as climate or economic models, to test hypotheses and predict future outcomes.
Related Reading
• Citing ChatGPT
• How To Find Academic Sources
• How To Cite AI In MLA
• How To Find Good Sources
• AI For Research Paper Writing
• Essay Sources
• AI In Academic Writing
• How To Get ChatGPT To Cite Sources
• Most Reliable Sources For Research
How AI Works in Research
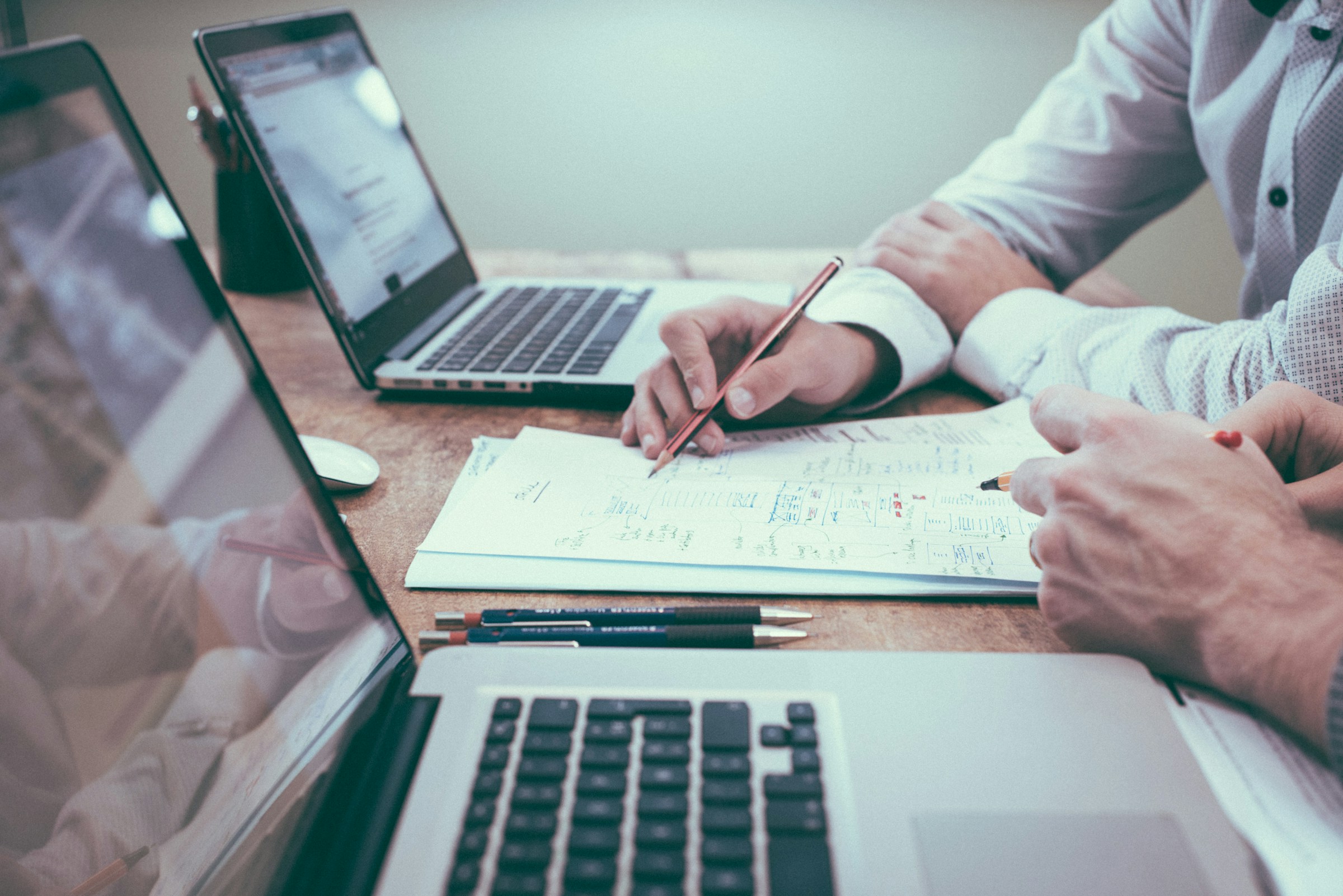
AI operates based on two primary components: machine learning and natural language processing (NLP).
Machine Learning: An Overview
Machine learning employs diverse algorithms, such as linear regression, decision trees, random forests, support vector machines, and neural networks, to learn from data and make predictions or decisions. These algorithms are trained on extensive datasets, enabling them to recognize intricate patterns, identify subtle trends, and make accurate inferences.
Through iterative training and refinement, the algorithm develops a model that can be used to analyze new data and generate insights. Once trained, the model's performance is rigorously evaluated using various metrics to assess its accuracy, precision, recall, and other relevant measures. This helps researchers understand the model's strengths and weaknesses and identify areas for improvement.
To optimize the model's performance, researchers often experiment with different hyperparameters, which are settings that control the learning process. This involves adjusting parameters such as learning rate, regularization strength, and network architecture to find the best combination for the specific research task.
Natural Language Processing (NLP): An Overview
NLP techniques empower AI to comprehend and interpret human language, including text and speech. These techniques allow machines to understand human language helpfully. For example, NLP can help AI analyze literature, write reports, or summarize research findings.
Fundamental Processes in AI for Research
Data Collection and Preparation
Researchers gather relevant data from various sources and preprocess it to ensure it's suitable for AI analysis. This often involves cleaning, formatting, and labeling the data to address inconsistencies and enhance quality.
Feature Engineering
AI algorithms require features or specific data characteristics from which to learn. Researchers may need to create or select relevant features that capture the essential information for the research question. This process can involve transforming raw data into more informative representations.
Model Selection and Training
The appropriate machine learning algorithm is chosen based on the research question, data type, and desired outcomes. The algorithm is then trained on the prepared data, iteratively adjusting its parameters to minimize errors and optimize performance.
Model Deployment and Inference
Once satisfied with the model's performance, it can be deployed to analyze new data and generate predictions or insights. The model can be integrated into research workflows or applications to automate tasks and support decision-making.
Continuous Improvement
AI is an evolving field, and researchers should continually evaluate and refine their models as new data becomes available and advancements in AI techniques are made. This includes updating the model's training data, exploring new algorithms, and addressing any limitations or biases that may be identified.
By understanding these fundamental components and processes, researchers can effectively leverage AI to enhance their research endeavors, uncover new insights, and drive innovation in their fields.
Benefits of Using AI for Research
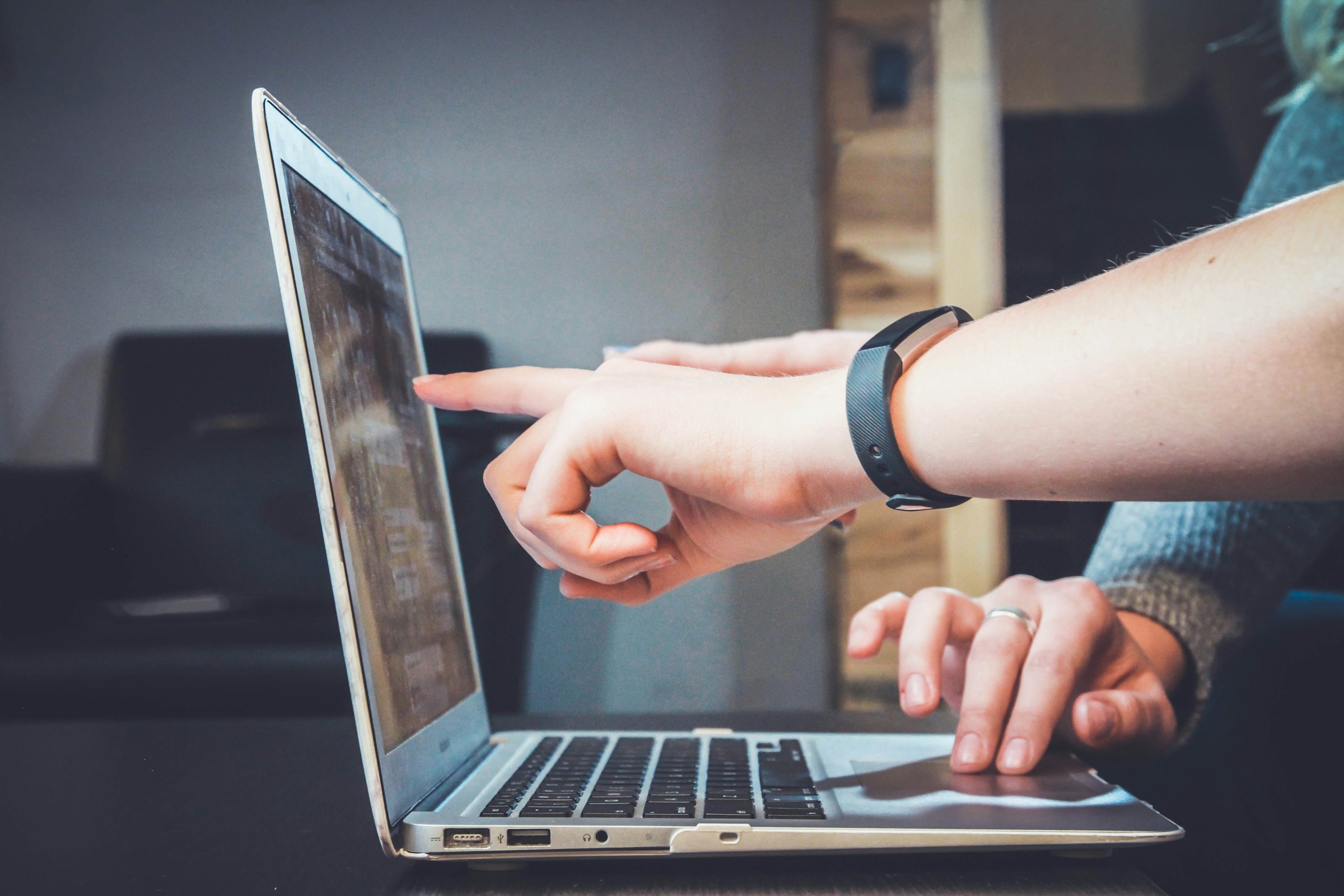
AI offers researchers many advantages, enabling them to enhance their work, accelerate discoveries, and gain deeper insights. Here are some of the key benefits:
Increased Efficiency
Automation of Repetitive Tasks
AI can automate routine tasks, such as data cleaning, preprocessing, and analysis, freeing researchers to focus on more complex and creative aspects of their work.
Faster Research Cycles
By automating time-consuming tasks, AI can significantly accelerate research cycles, enabling researchers to achieve results more quickly.
Improved Productivity
AI can help researchers increase their productivity by streamlining their workflows and reducing the time spent on mundane tasks.
Improved Accuracy
Reduced Errors
AI algorithms can analyze data more precisely than humans, reducing the risk of errors and biases.
Enhanced Data Quality
AI can help identify and correct errors in data, improving its quality and reliability.
Increased Trustworthiness
By using AI to analyze data, researchers can increase the trustworthiness of their findings and reduce the likelihood of making mistakes.
Enhanced Insights
Uncovering Hidden Patterns
AI can uncover hidden patterns and trends in data that may not be apparent to human researchers.
Generating New Hypotheses
AI can stimulate researchers to explore novel research directions by generating new hypotheses based on data analysis.
Deeper Understanding
AI can help researchers gain a deeper understanding of their research topics by providing new perspectives and insights.
Accelerated Discovery
Faster Innovation
AI can help researchers identify new research directions and accelerate the pace of scientific discovery.
Breakthroughs
By analyzing large datasets and identifying patterns, AI can contribute to breakthroughs in various fields, including medicine, materials science, and climate research.
Competitive Advantage
AI can give researchers a competitive advantage by enabling them to make discoveries and publish their findings more quickly than their competitors.
Improved Collaboration
Enhanced Communication
AI-powered tools can facilitate collaboration among researchers, enabling them to share data, insights, and resources more effectively.
Interdisciplinary Research
AI can foster multidisciplinary research by breaking down silos between different fields and enabling researchers to work together on complex problems.
Increased Impact
By collaborating with other researchers and leveraging AI tools, researchers can increase the impact of their work and make a more significant contribution to their field.
Ethical Considerations
While AI offers numerous benefits, the ethical implications of its use in research must be considered. Researchers must ensure that AI is used responsibly and ethically, avoiding biases and ensuring data privacy.
Critical Characteristics of AI for Research
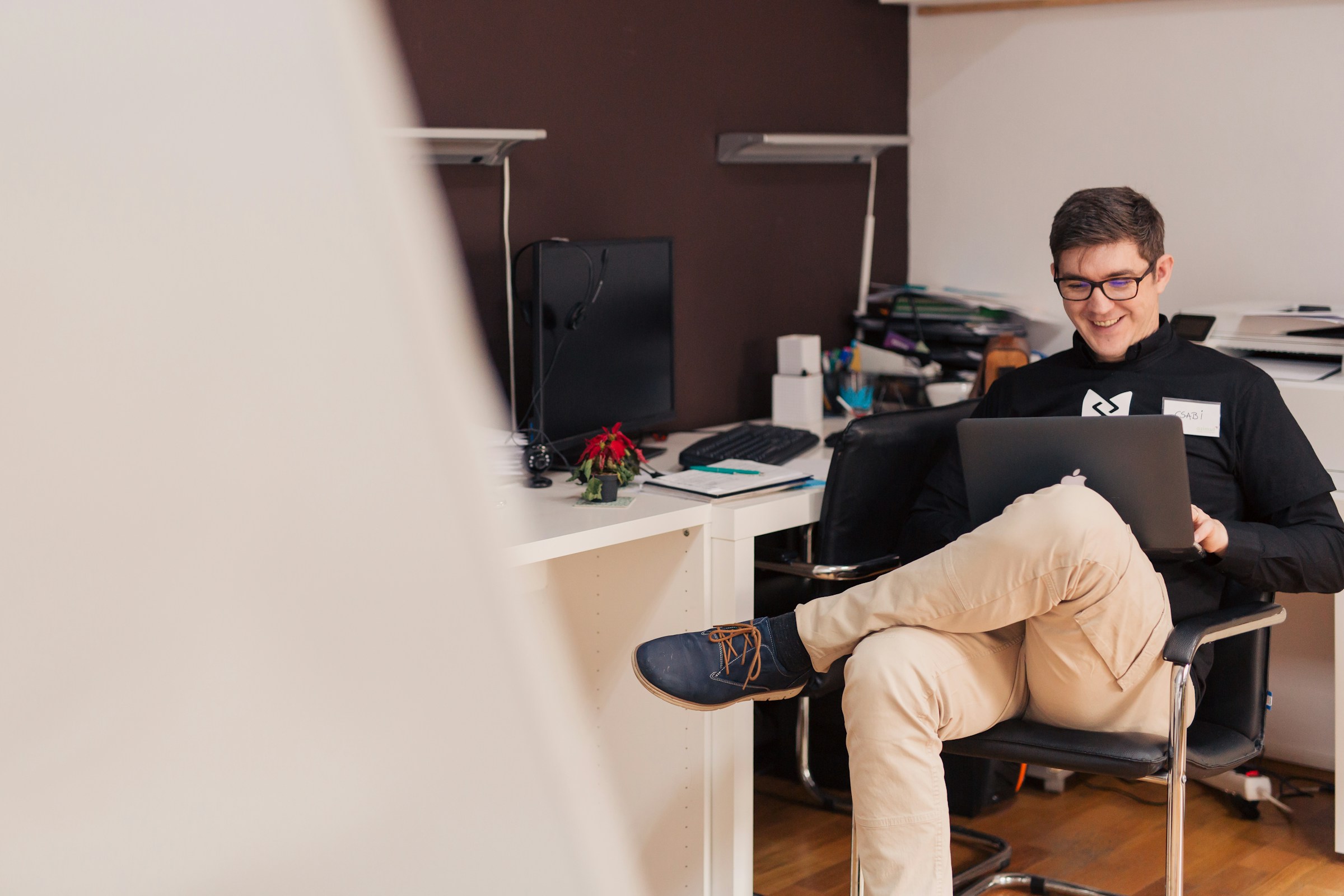
Natural Language Processing and Its Role in Research
AI for research is characterized by several key features that enable it to enhance the capabilities of researchers and drive innovation. These characteristics include:
Natural Language Processing (NLP)
Text AnalysisNLP allows AI to understand and interpret human language, including text and speech. This enables researchers to analyze large volumes of textual data, extract essential information, and identify trends and patterns.
Sentiment AnalysisNLP can determine the sentiment expressed in text, such as whether it is positive, negative, or neutral. This is valuable for understanding public opinion, social media trends, and customer feedback.
Information ExtractionNLP can extract specific information from text, such as named entities (e.g., people, organizations, locations) or critical facts. This is useful for building knowledge graphs and conducting literature reviews.
Machine Learning
Algorithm DiversityAI for research leverages many machine learning algorithms, including linear regression, decision trees, random forests, support vector machines, and neural networks. Each algorithm has strengths and weaknesses, making it suitable for different research tasks.
Data-Driven Learning Machine learning algorithms learn from data, enabling them to improve their performance over time. This allows AI to adapt to new data and changing research contexts.
Predictive Modeling Machine learning can be used to build predictive models that forecast future trends or outcomes. This is valuable for research in finance, healthcare, and climate science.
Data Analysis and Visualization
Data Exploration: AI can help researchers explore large, complex datasets to identify patterns, trends, and anomalies.
Data Visualization: AI can generate visualizations of data, such as charts, graphs, and maps, making it easier to understand and communicate research findings.
Insights Generation: By analyzing data and generating visualizations, AI can help researchers discover new insights and uncover hidden relationships.
Automation
Task Automation: AI can automate repetitive and time-consuming tasks, such as data cleaning, preprocessing, and analysis. This frees researchers to focus on their work's more complex and creative aspects.
Workflow Optimization: AI can help researchers optimize workflows by automating tasks and streamlining processes. This can increase efficiency and productivity.
Scalability: AI can handle large-scale datasets and complex research problems, making it suitable for various research applications.
Adaptability
Continuous Learning: AI algorithms can continuously learn and improve as they are exposed to new data. This allows them to adapt to changing research contexts and remain relevant over time.
Flexibility: AI can be applied to various research domains and customized to meet the specific needs of different researchers.
Innovation: AI can drive innovation by enabling researchers to explore new research directions and develop novel approaches to problem-solving.
Steps to Use AI for Research
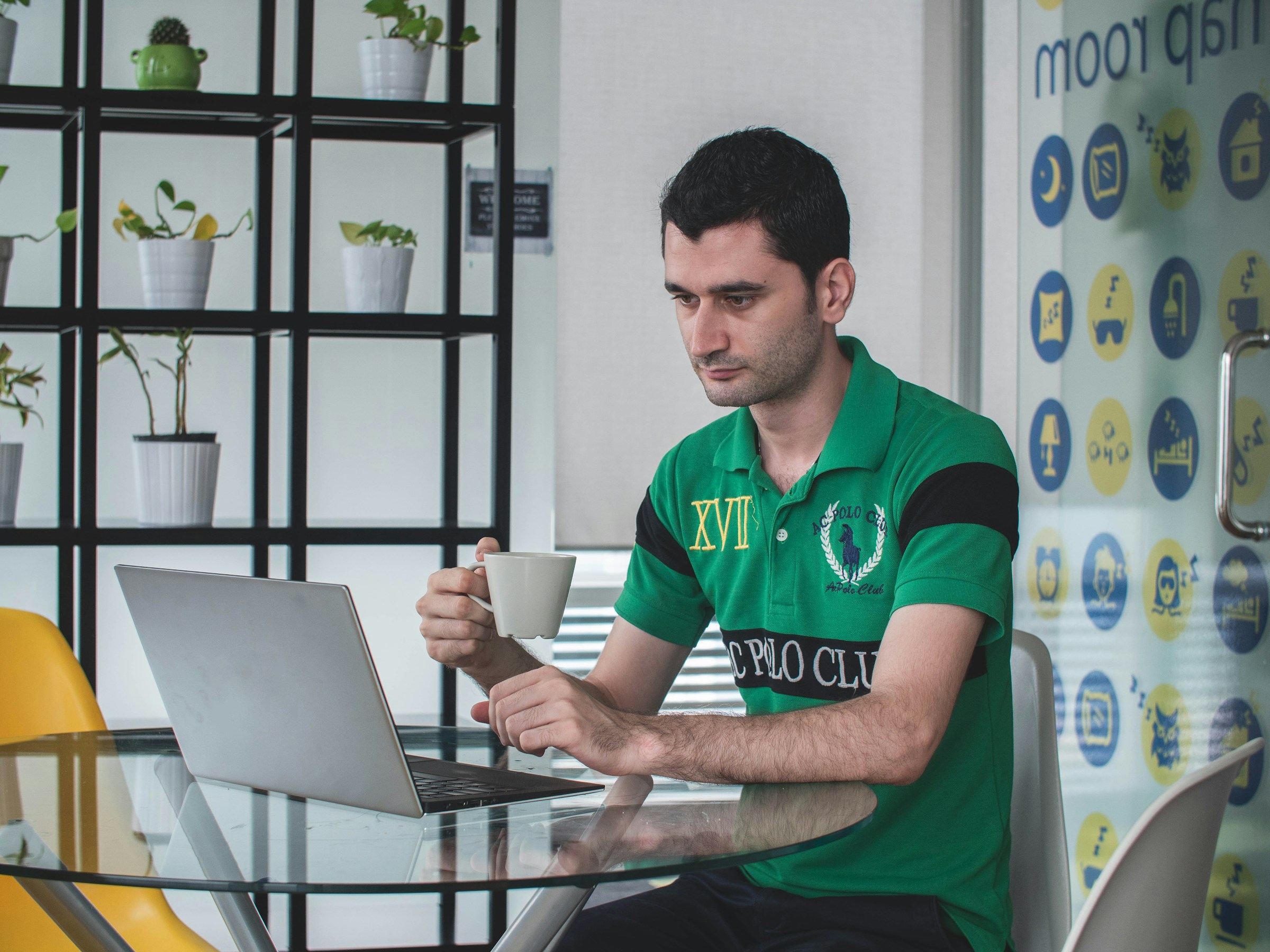
Pinpoint Your Research Question or Topic
The first step in leveraging AI for research is identifying your research question or topic. This ensures your AI tools and techniques align with your specific goals. Be as detailed as possible. Consider the particular questions you want to answer or the problems you want to solve. Break down your research question into smaller, more manageable subquestions. This will guide your analysis and ensure your research is focused and well-defined.
Gather Relevant Data
Next, focus on collecting the data you will use to fuel your AI research. This will likely involve gathering information from various sources, including existing datasets, experiments, simulations, or surveys. Ensure the data is relevant, accurate, and representative of your research question. Also, consider the quality and quantity of the data you need to achieve your research objectives. A sufficient amount of high-quality data is essential for training and evaluating AI models.
Choose Appropriate AI Tools
Once you have a straightforward research question and relevant data, it is time to select your AI tools. Look for platforms that will be suitable for your specific research needs. Consider factors such as the type of data you are working with, the complexity of your analysis, and your level of technical expertise. Explore the capabilities and limitations of different AI tools to find the best fit for your research. Also, factors like ease of use, scalability, and integration with other tools should be considered when selecting.
Prepare Your Data
Before applying AI techniques to your research, you must prepare your data. This involves cleaning, preprocessing, and formatting the information to make it suitable for AI analysis. Remove missing values, handle outliers, and address inconsistencies in the data to ensure its quality and reliability. Convert data into an appropriate format, such as CSV, JSON, or SQL. You may also consider feature engineering techniques to create new features that may be more informative for your analysis. This can involve transforming raw data into more meaningful representations that capture the underlying patterns and relationships.
Apply AI Techniques
Next, it is time to apply the AI techniques that will help you analyze your data. Choose the approaches based on your research question and the nature of your data. Consider factors such as the complexity of your analysis, the desired level of accuracy, and the interpretability of the results. Experiment with different AI techniques to find the best approach for your research. Consider using ensemble methods, which combine multiple models to improve performance and reduce overfitting.
Interpret and Analyze Results
Once you have applied your AI techniques, examine the output of your analysis to identify patterns, trends, and insights. Use your domain knowledge to interpret the results and draw meaningful conclusions. Be sure to consider the limitations of your AI analysis and the potential for biases or errors. Validate your findings through additional analysis or experiments to ensure their reliability and credibility.
Refine and Iterate
Your research will likely benefit from an iterative approach. Based on your initial analysis, refine your strategy and iterate as needed. Collect additional data, if necessary, to improve the quality and quantity of your analysis. Adjust your AI techniques or explore alternative methods if you encounter challenges. Continuously evaluate your research progress and make adjustments to ensure your research is on track and achieving your desired outcomes.
Essential Tools and Platforms for AI Research
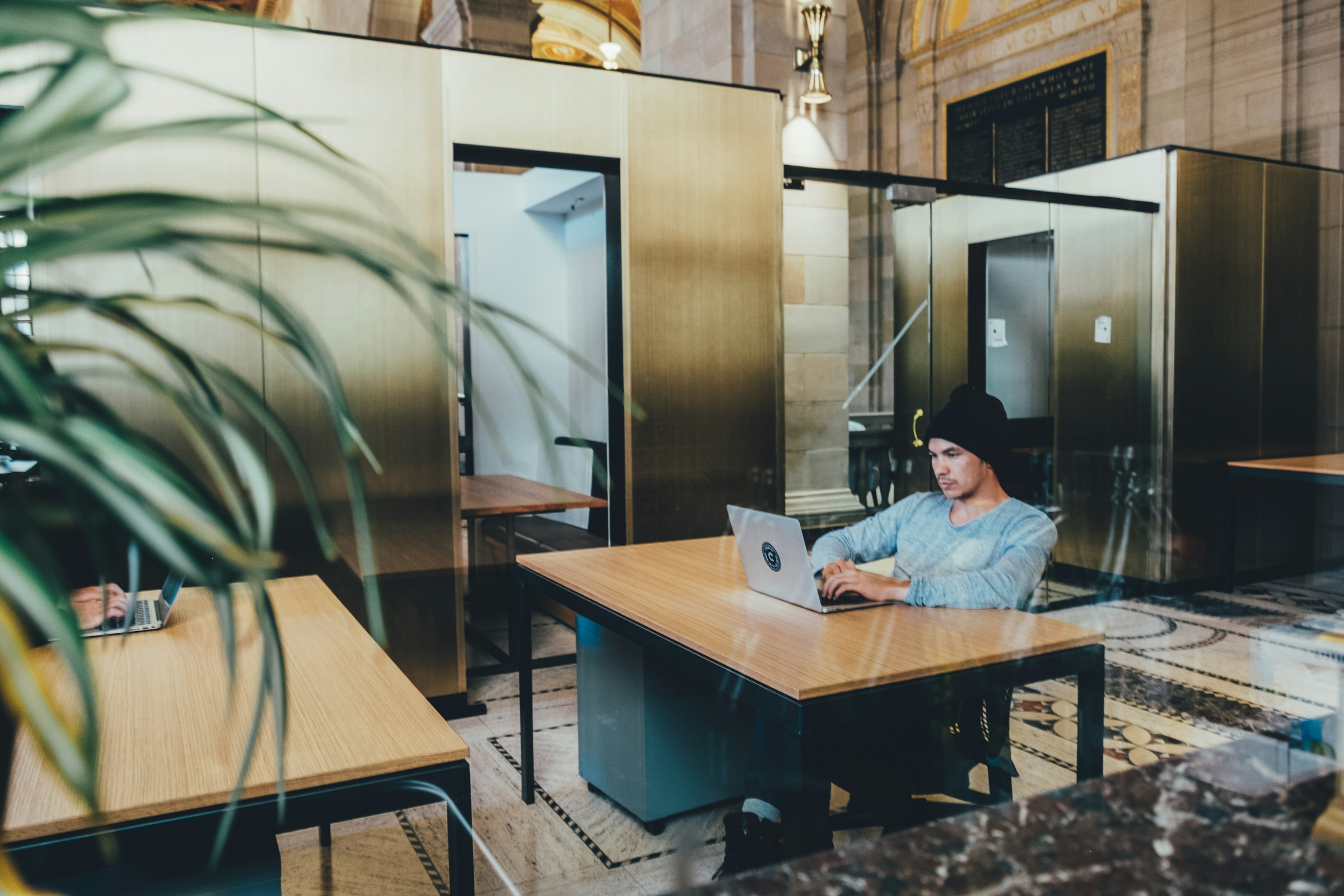
Data Science Platforms
Explore Tools Like Jupyter Notebook, Google Colab, and Kaggle to Help with Research AI Jupyter Notebook is a famous interactive computing and data science tool that allows researchers to combine code, visualizations, and text in a single document. This makes it easy to experiment, iterate, and share their work.
Google Colab is a free cloud-based platform that provides access to GPUs and TPUs, making it ideal for computationally intensive research tasks. Colab eliminates the need for researchers to invest in expensive hardware, enabling them to focus on their research without worrying about infrastructure constraints. Kaggle is a platform for data science competitions and collaboration. It offers a vast repository of datasets and pre-trained models. Researchers can participate in competitions to test their skills, learn from others, and access valuable resources.
Machine Learning Libraries
Get Familiar with TensorFlow, PyTorch, and Scikit-Learn to Research AI. Open-source machine learning libraries are crucial for researchers looking to leverage AI. TensorFlow is an open-source platform developed by Google for machine learning. It provides a flexible and scalable framework for building and training models. It offers many features, including support for distributed training, model deployment, and integration with other tools. PyTorch is another popular open-source machine learning framework. It is known for its ease of use and dynamic computational graph.
PyTorch's flexibility and intuitive interface make it a popular choice for researchers new to machine learning. Scikit-learn is a Python library for machine learning that offers a comprehensive set of algorithms and tools for data preprocessing, modeling, and evaluation. It provides a user-friendly API and extensive documentation, making it accessible to researchers of all levels.
Natural Language Processing Libraries
Learn About NLTK, spaCy, and Gensim for Text-Based AI Research When it comes to AI for research, text data can be one of the most important types to analyze. Natural language processing (NLP) allows researchers to crunch numbers on text data to uncover patterns, trends, and actionable insights.
NLTK is a Python library for natural language processing that provides a comprehensive set of tools for tasks such as tokenization, stemming, tagging, and parsing. NLTK is a valuable resource for researchers working with textual data, enabling them to extract meaningful information and insights. spaCy is another Python library for NLP known for its speed and efficiency, making it suitable for large-scale text analysis.
spaCy offers a range of features, including named entity recognition, part-of-speech tagging, and dependency parsing. Gensim is a Python library for topic modeling and document similarity. Gensim helps analyze extensive collections of text data and provides tools for discovering latent topics in text data, identifying similar documents, and exploring semantic relationships.
Cloud Computing Platforms
Access Powerful Tools Through AWS, Google Cloud, and Microsoft Azure AI for research can require significant computational power, especially when working with large datasets or complex models. Cloud computing platforms provide flexible and scalable resources for researchers to leverage. Amazon Web Services (AWS) is a leading cloud computing platform that offers a wide range of services, including EC2 instances for computing power, S3 for storage, and SageMaker for machine learning.
AWS provides a scalable and flexible infrastructure for researchers, allowing them to access the resources they need to conduct their research without the overhead of managing their hardware. Google Cloud Platform (GCP) is another primary provider offering services similar to AWS, specialized data analytics, and machine learning tools. GCP's BigQuery service is handy for researchers who need to analyze large datasets efficiently.
Microsoft Azure is a cloud platform from Microsoft that provides a comprehensive suite of services for businesses and researchers. Azure offers various tools and services for data science and machine learning, including Azure Machine Learning Studio and Azure Databricks.
Visualization Tools
Create Visuals to Make Your Research AI More Understandable Matplotlib is a Python library for creating static, animated, and interactive visualizations. Matplotlib provides a flexible and customizable framework for creating a wide range of plots and charts. Seaborn is a Python library built on Matplotlib that provides a high-level interface for creating attractive and informative visualizations.
Seaborn offers a variety of pre-built styles and themes, making it easy to create visually appealing plots. Plotly is a Python library for building interactive visualizations that can be embedded in web pages or shared as standalone plots. Plotly offers various visualization types, including charts, graphs, maps, and dashboards.
Version Control Systems
Using Git and GitHub to Collaborate with Other Researchers AI for research often involves collaboration between multiple data scientists, especially when working on large projects. Git is a popular version control system that allows researchers to track changes to their code, collaborate with others, and revert to previous versions if necessary.
Git is essential for managing research projects, especially when working in teams or collaborating. GitHub is a web-based platform hosting Git repositories that provides additional features such as issue tracking, code review, and project management. GitHub offers a convenient way to share and collaborate on research projects and access to a vast community of developers and researchers.
Start Writing For Free With Jotbot Today!
Jotbot is your personal document assistant and source finder. Jotbot does AI note-taking, AI video summarizing, and AI citation/source finder; it writes AI outlines for essays and even writes entire essays with Jotbot’s AI essay writer.
Join 500,000+ writers, students, teams, and researchers worldwide to write more, write better, and write faster with Jotbot's AI writing assistant. Write more intelligently, not harder, with Jotbot's AI writing assistant. Start finding sources for free with Jotbot's source finder today. Sign in with Google and get started in seconds.
Related Reading
• Reference Finder
• Best AI For Research
• How to Cite AI
• AI For Literature Review
• Websites Like Google Scholar
• AI That Cites Sources
• Best Academic Search Engines
• Best AI Tool For Research
• Academic Research Software
• How To Use Chat GPT For Research
• Best Research Tools For Students
10 Things to Keep in Mind When Using AI for Research
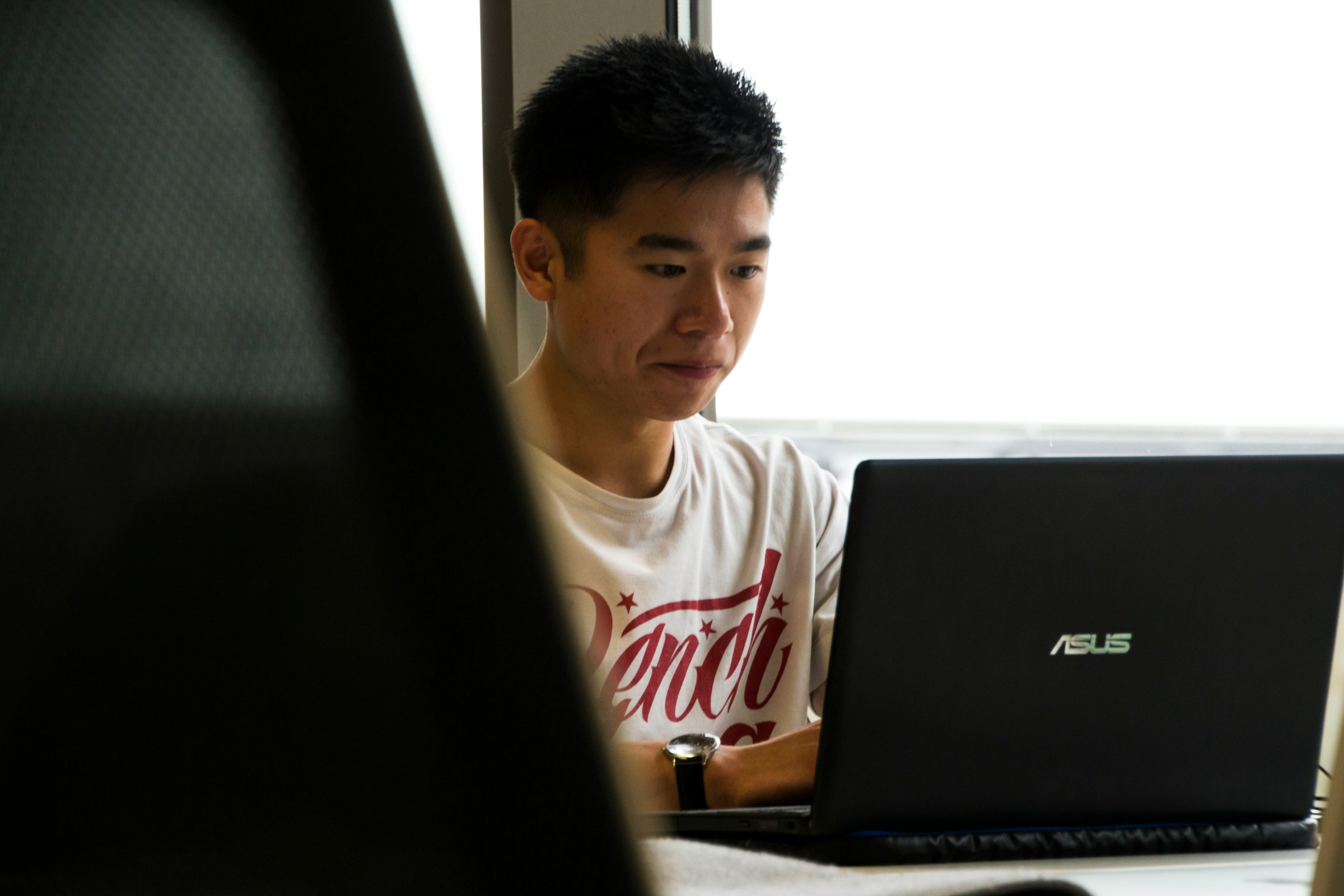
1. Data Quality: The Foundation of Reliable AI Research
The data you use for AI research ultimately determines the quality of your findings. Ensure that the data you use for your research is accurate, reliable, and representative of your study population. Poor quality data can lead to inaccurate results and misleading conclusions. Thoroughly clean and preprocess your data to remove errors, inconsistencies, and missing values. This will improve the quality of your analysis and enhance the reliability of your results.
2. Bias: Be Aware of AI’s Potential for Discrimination
AI is not free of bias. AI can perpetuate and even exacerbate human biases, particularly when it comes to sensitive topics. Algorithm bias can lead to unfair or discriminatory results, undermining the credibility of your research. Data bias can distort your research findings. Therefore, ensure that your data is representative of the population you are studying and does not contain any biases that could distort your results.
3. Ethical Considerations: Use AI Responsibly
Using AI for research raises ethical considerations that must be addressed. First, respect the privacy of individuals and ensure that you have obtained appropriate consent before collecting and using their data. Second, be mindful of intellectual property rights and avoid infringing on the rights of others. Finally, use AI responsibly and ethically, avoiding harmful or discriminatory applications.
4. Limitations of AI: Don’t Forget About Human Oversight
AI is not a substitute for human judgment and expertise. Using AI to support your research is essential rather than relying solely on its output. Some AI models can be difficult to interpret, making understanding how they arrive at their conclusions challenging. This can limit the transparency and credibility of your research.
5. Collaboration: Don’t Go It Alone
AI isn’t a magic bullet that will solve all your research problems. Collaborate with experts in your field to ensure you use AI effectively and correctly interpret the results. Additionally, seek the advice of AI experts to help you select the appropriate tools and techniques for your research.
6. Continuous Learning: Stay Ahead of the Curve
Stay up-to-date on the latest developments in AI and research methods. This will help you use AI tools more effectively and stay ahead of the curve in your field. Be willing to experiment with different AI techniques and approaches. Continuously evaluate your research progress and adjust as needed to improve your results.
7. Transparency: Don’t Hide Your Methods
Be transparent about your research methods and the use of AI tools. This includes disclosing the limitations of your AI analysis and the potential for biases. Make your research results reproducible so others can verify and validate your findings. This involves detailed documentation of your methods, data sources, and AI tools.
8. Accessibility: Ensure AI Tools Are Equitable
Consider the accessibility of AI tools and ensure that they are inclusive and equitable. This includes making tools accessible to people with disabilities and ensuring they are not biased against certain groups.
9. Impact: Reflect on the Societal Implications of Your Work
Reflect on the potential impact of your research and the use of AI tools. Consider the social, economic, and environmental implications of your work. Strive to use AI for positive change and to address critical societal challenges.
10. Sustainability: Use AI Responsibly
Use AI tools and techniques that are resource-efficient and sustainable. This includes minimizing the environmental impact of your research. Also, the long-term viability of AI technologies and their potential to contribute to sustainable development should be considered.
Use Jotbot to Supercharge Your Research Process
Jotbot is a versatile AI-powered tool designed to streamline your research process and enhance the quality of your work. By leveraging Jotbot's capabilities, you can:
Streamline Your Research Process
AI Note-Taking: Jotbot's automatic transcription feature saves you time and effort by converting audio and video recordings into text. This allows you to focus on your research without worrying about manual note-taking.
AI Video Summarizing: Jotbot's ability to summarize key points from videos ensures that you extract the most relevant information efficiently. This is particularly useful when dealing with long or complex videos.
AI Citation/Source Finder: Jotbot's influential citation and source finder automates finding and citing relevant sources, ensuring your research is well-supported and credible. This eliminates the time-consuming task of manually searching for and formatting citations.
Improve Your Writing
AI Outlines for Essays: Jotbot can help you create structured outlines for your essays, making organizing your thoughts and ideas more accessible. Jotbot can help you write more effectively and efficiently by providing a clear framework.
AI Essay Writer: Jotbot's AI essay writer can assist you in writing entire essays, providing suggestions, and improving your writing style. This can be particularly helpful when struggling with writer's block or needing to produce a high-quality essay quickly.
Access a Vast Knowledge Base
Source Finder: Jotbot's source finder gives you access to a vast knowledge base of information and citations. This can help you discover relevant research papers, articles, and other resources that support your research.
Join a Community of Researchers
Collaboration: Connect with other researchers and writers who use Jotbot to share ideas, collaborate on projects, and receive support. Being part of a community can provide valuable insights and networking opportunities.
By leveraging Jotbot's capabilities, you can significantly improve your research efficiency, productivity, and the quality of your work. Jotbot is a valuable tool for researchers at all levels, from students to seasoned professionals.
Write Smarter With Jotbot's Source Finder — Start Writing for Free Today
Researching and writing essays can be a long and tedious process. Finding credible sources, taking notes, writing an outline, and drafting your paper are challenging. Fortunately, Jotbot can help you with every step of this process, especially when finding sources.
Jotbot’s source finder lets you enter a prompt about your essay and find relevant sources to help you write your paper. You can even get video sources! Jotbot will summarize the sources to quickly get the necessary information without reading through every article. This can save you hours of research time.
Related Reading
• Elicit AI
• Scholarcy AI
• Scisummary
• AI Research Tools
• Sourcely
• Consensus AI Tool
• Mendeley Alternatives
• Cite This For Me Alternative
• Scholarly Sources Examples
• Academic Sources Examples
• How to Find Scholarly Sources
• List of Scholarly Sources
• Examples of Peer Reviewed Sources
• How to Cite a Book
• How to Cite an Article
• How to Cite
• How to Cite a PDF
• How to Cite Multiple Authors MLA
• How to Cite a Website in Text
• How to Cite a Lecture
• How to Cite ChatGPT
Write more, better, faster.
Your personal AI document assistant